Artificial intelligence (AI) has made its way into every business process, with supply chain management being no exception. Forty-one percent of business leaders are making AI a key part of their supply chain innovation strategy, with a third already leveraging it for data visibility, 29% for quality control, and 26% for labor optimization. An impressive 72% of leaders are also deploying Generative AI in their supply chains.
That said, the adoption of AI in supply chain management has been a steep climb. Primarily because it requires transformations in other areas like cloud infrastructure, data management, and cybersecurity, leading to lower ROI.
So how and where can SCMs realize ‘quick wins’ from AI investments? Our bet is on deploying gen AI with retrieval-augmented generation, plus being precise with ML use case prioritization.
What is RAG in AI?
Retrieval-augmented generation (RAG) is a generative AI framework that combines natural language generation with information retrieval to produce more accurate and context-aware responses.
Instead of relying solely on its pre-trained “knowledge”, a large language model (LLM) actively searches a knowledge base or external documents to retrieve relevant information in real time. The retrieved content is then used to ground the generated output, improving factual accuracy and reducing model hallucinations.
The original credit for this framework goes to researchers from META AI. They came up with the idea of “packaging” retrieved information into a connected vector database — a specialized data store that can host high-dimensional vector data derived from text, visuals, or audio as mathematical vectors. The vector format enables LLMs to access new knowledge in a matter of seconds and produce more relevant outputs.
With RAG, general-purpose LLMs like GPT and Claude can be adapted to domain-specific contexts without incurring massive training costs. That’s handy given that 46% of CIOs say lack of predictability in pricing is a key blocker to gen AI implementation.
RAG-powered LLMs quickly became a staple for all sorts of knowledge-related tasks in supply chain management (SCM) and beyond. Yet, it’s just only one “flavour” of AI in supply chain management.
Other AI Technologies Used in Supply Chain Management
AI is an umbrella term for a large number of sub-technologies actively deployed for supply chain digitization and optimization. Gartner ranked “achievement of capability of AI” as the key future driver of supply chain management, ahead of factors like ESG regulations and trade tensions.
In particular, SCM leaders are actively investing in the following technologies:
- Predictive analytics tools use historical and real-time data to forecast future demand, identify potential disruptions, and optimize operational planning.
- Decision Intelligence (DI) systems augment and automate decision-making with statistical decision modeling, analytics, and explainable AI.
- Augmented Connected Workforce (ACWF) solutions enhance frontline workforce productivity, improve on-the-spot decision-making, and accelerate onboarding in logistics operations.
- Agentic AI systems can autonomously execute business tasks like inventory optimization or dynamic shift scheduling to improve operational efficiency.
- Intelligent simulation tools combine traditional simulation methods with AI-enabled predictive and decision-making capabilities for advanced supply chain scenario planning.
- Computer vision systems enable real-time analysis of images and video for quality control, warehouse automation, and safety monitoring.
- Digital twins use 3D modeling, IoT data, and predictive algorithms to build virtual models of physical supply chain systems for real-time optimization and simulations.
- AI-based Route Optimization (AIRO) algorithms bring real-time adaptability to route planning based on traffic, weather, and delivery constraints to reduce costs and emissions.
- Intelligent Document Processing (IDP) systems automate data extraction, validation, review, and sharing from supply chain documents like COAs and invoices.
Generally, AI is a major line in SCMs technology budgets, although many are also looking into other areas like robotics and multimodal UI.
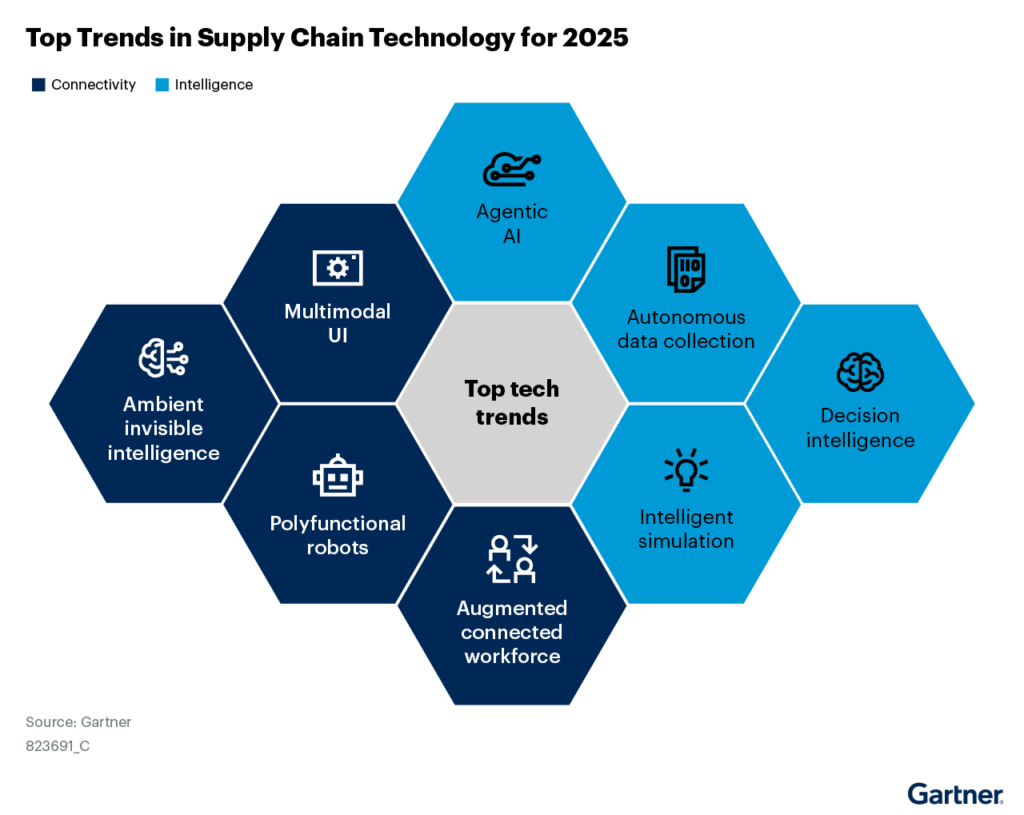
Source: Gartner
The Most Feasible and Valuable AI Use Cases in Supply Chain Management
Supply chain leaders have a natural caution towards new technologies. Three-quarters agree that their sector has been slow to embrace digital innovation. To an extent, this can be explained by the underlying workflow complexity and complex compliance landscape. However, neither are good enough “excuses” to pass on the benefits of AI in supply chain optimization.
To maximize the gains and minimize the risks, SCMs should strategically approach AI use case selection and validation. Edvantis team did some grunt work for you, sifting through dozens of AI applications in supply chain management to pinpoint those with fast ramp-up time, minimal operational disruption, and proven ROI.
Decision Intelligence
Volatility is the new baseline. About half (47%) of global supply chain leaders see their operations as vulnerable to disruption. Shifting regulations and tariffs, new sustainability mandates, and geopolitical unrest, among other factors, put extra pressure on SCMs.
Many are responding by rethinking their sourcing strategies, moving away from globalization in favor of more localized supplier networks. Onshoring and nearshoring are no longer fringe strategies. They’ve become essential for balancing cost efficiency with risk mitigation.
AI-powered decision intelligence systems are helping leaders make the right choices. By combining decision modeling with machine learning, DI enables organizations to gain operational foresight: 92% of executives surveyed by LeanDNA say that AI-driven insights are essential for predicting and preventing disruptions. What’s more, the majority (87%) expect to see positive returns from such AI and ML investments in the next year or two.
There are indeed proven grounds for such optimism based on the early adopters’ outcomes.
SPAR Austria, a leading food retailer with over 1,500 stores, recently partnered with Paiqo and Microsoft to enhance its demand forecasting using Azure-based AI solutions. The new system improved SPAR’s demand forecasting accuracy by 90%, allowing the company to optimize product order quantities per store to cut food waste and prevent stockouts.
Taiwan’s Foxconn, a global manufacturing partner for companies like Apple, Cisco, Nokia, and Sony, among others, recently launched a domain-specific LLM optimized for traditional Chinese and Taiwanese languages. According to the spokesperson, the FoxBrain System supports decision-making across data analysis, document collaboration, reasoning, and even code generation, bringing end-to-end intelligence to the company’s manufacturing and supply chain operations.
By embedding decision intelligence into their supply chains, companies move beyond basic analysis toward a more adaptive, forward-looking model. Instead of relying on static dashboards or backward-looking KPIs, they gain the ability to simulate outcomes, weigh trade-offs, and act with precision, even amid prolonged economic uncertainty.
Customer Relationship Management
Just like in other sectors, customer experience (CX) has become front and center in the logistics and transportation sectors. Over 90% of companies admit that customers’ expectations of service offerings and service quality have risen significantly. Most clients expect real-time tracking visibility, faster delivery timelines, and more proactive issue resolution.
Yet, many companies grapple with persistent labor shortages, fragmented data systems, and limited visibility across the full logistics lifecycle. These factors affect inquiry investigation time and limit businesses’ ability for personalized engagement.
Generative AI agents are emerging as “the connective tissue”, bridging gaps between siloed systems and overburdened teams. By automatically retrieving relevant data, triggering workflows, and generating human-like responses, GenAI tools can drastically improve both responsiveness and service quality.
The Dutch postal service, PostNL, recently launched SuperTracy, an agent for tracking shipment statuses. Designed as a multi-agent system with a RAG, SuperTracy can autonomously handle customer inquiries about parcel tracking, using internal knowledge bases to generate accurate responses. Beyond improving customer satisfaction, the tool has also streamlined internal knowledge management, reducing the burden on service teams and improving resolution times across the board.
DHL Supply Chain gives another example of a successful Gen AI deployment for CX. The logistics company launched an AI-powered sales copilot to streamline customer proposal development. The tool analyzes customer requirements, industry data, and historical deal parameters to generate more accurate and personalized proposals in a beat. With data prep out of the way, DHL’s sales team can focus on solving complex customer challenges and building stronger relationships.
Supply Chain Simulations
Supply chain managers are juggling more priorities than ever. They need to keep lean costs, optimize inventory levels, minimize disruption, and meet sustainability targets. These competing goals often pull operations in different directions, making it harder to strike the right balance between efficiency and resilience.
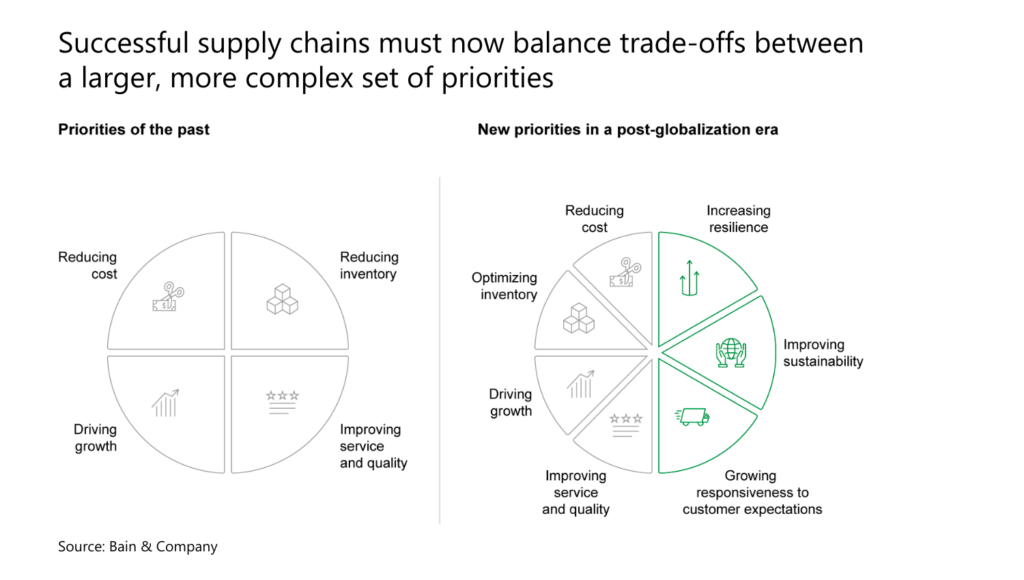
Source: Bain
This is where AI-driven supply chain scenario simulations can make a difference. By modeling thousands of what-if situations, from supplier disruptions to shifts in demand, AI allows you to test new approaches before committing to them. For example, evaluate new warehouse layouts, alternative suppliers, or new shipment routes. By running virtual simulations, you can reduce the high stakes of making costly missteps.
Siemens is among the pioneers of this movement. To diversify its supplier risks, the company deployed a collection of AI tools for supply chain planning. Through collaboration with Supplyframe, Siemens deployed an advanced risk analysis system, which gives the company real-time intelligence into the electronics supply chain, allows tracing component availability, and runs advanced cost analysis. These features are now part of the Siemens Xcelerator digital twin platform.
One of Siemens’ customers, Radeberger Gruppe, a major brewery group in Germany, also sees great value in AI-powered simulations. “Using a digital twin gives us the big picture of the entire breadth and depth of our brewery group’s supply chain,” shared Emil Wagner, Director of Digitalisation, Processes and Projects at Radeberger Gruppe. Radeberger Gruppe gained real-time visibility into its logistics operations and the ability to simulate future scenarios with remarkable precision. “This allowed us to grow the capacity of our existing production infrastructure well into the double digits,” he adds.
AI simulations enable proactive decision-making and continuous optimization. They give supply chain leaders the power to test the future before it arrives and build smarter, stronger, more responsive operations.
Risk Management
SCMs face an expanded risk radar — geopolitical tensions, supplier instability, regulatory changes, cyber threats, and climate impacts are just the tip of the iceberg. Traditional risk management systems weren’t built for this level of complexity. So, they struggle to process the vast, dynamic variables influencing global operations.
Predictive AI models, combined with conversational AI interfaces, are now being tested as an alternative. Unlike traditional BI tools, such models can process both structured and unstructured data — from news reports and financial filings to sensor feeds and logistics updates — to surface early warning signs. Generative AI then takes this one step further by creating scenario simulations, dynamic contingency plans, and tailored risk-mitigation strategies, enabling teams to shift from reactive problem-solving to proactive resilience.
The US Defense Logistics Agency (DLA) has already deployed several AI models for risk management. AI models help DLA forecast demand spikes, predict supply chain bottlenecks, and recommend alternative suppliers to minimize disruption. Another model was trained to flag high-risk vendors — those supplying non-compliant, overpriced, or even counterfeit items — so procurement teams can course-correct. DLA Aviation unit, in turn, uses AI to identify opportunities for strategic bulk purchasing, increasing supplier interest and stock availability while reducing long-term costs.
Meanwhile, Capgemini built a gen AI risk management platform for the automotive sector. The tool ingests vast amounts of multilingual, multi-source, unstructured data to power supply chain risk assessments. Key features include:
- Supplier mapping for deep visibility across multiple tiers of the supply chain
- Risk and sustainability scoring to guide better supplier selection and allocation decisions
- Real-time monitoring, delivering actionable insights into disruptions as they unfold
When suppliers span borders and industries, predictive risk management becomes mission-critical. By making risk visible and manageable in real-time, AI allows SCMs to ingrain greater resilience and agility into their operations.
Route Optimization
With fuel costs on the rise, greater push for sustainability, and customer demands for faster logistics, SCMs need better capabilities for route optimization. Every mile saved can significantly impact both the bottom line and ESG ratings.
AI-powered fleet management solutions bring a roster of great capabilities:
- Dynamic route planning based on real-time traffic, weather, road closures, and delivery schedules
- Multi-stop delivery optimization that sequences deliveries to minimize time and fuel consumption
- Fleet load and capacity balancing, ensuring vehicles are used efficiently based on cargo size and urgency
- Sustainability and emissions optimization, with algorithms selecting eco-friendly routes to lower carbon footprints
- Cost-to-serve analysis, where AI factors in fuel, labor, and time to prioritize cost-effective paths
These features don’t just reduce operational waste — they unlock serious savings. When UPS rolled out its On-Road Integrated Optimization and Navigation (ORION) platform, it saved the company $320 million in the first year. With 35,000 of its 55,000 drivers using the system, fuel consumption went down by 10 million gallons. Today, ORION continues to save the company heaps in mileage costs.
In addition, AI route optimization (AIRO) systems lowered labor costs because drivers are on the road less. As are the vehicles which means less wear and tear, leading to lower maintenance bills.
Although, the biggest advantage of AIRO is dynamic route optimization. Freight company ArcBest recently launched a “cognitive” system for city route planning. The tool fuses traditional ML with advanced technologies like natural language processing and vision analytics to predict the best routes and adapt delivery sequences in real time. Rolled out in 2013, the system has already saved ArcBest over $13 million in operating costs.
In the maritime sector, Hyundai’s OceanWise platform showcases the global potential of AI-driven routing. Using big data and AI algorithms, the system recommends optimal shipping paths that minimize fuel consumption and greenhouse gas emissions. In real-world tests, OceanWise produced an average fuel saving of 5.3%, translating to KRW 350 million (around $240,000) in annual savings per vessel.
The takeaway? From trucks to cargo ships, AIRO systems cut costs, reduce emissions, and make logistics more adaptable to real-world conditions.
Compliance Management
Supply chain managers often face a barrage of (sometimes conflicting) regulations — evolving trade laws, new ESG mandates, anti-corruption rules, and data privacy standards. Similarly, compliance is no longer a box-ticking exercise because regulators keep a watchful eye. Many multinationals face a steep 5% of their worldwide turnover fine if they fail to comply with the EU Corporate Sustainability Due Diligence Directive by 2027.
AI can help stay in sync with the current regulations and be ahead of the curve when it comes to changes. Thanks to superior data processing capabilities, such systems can digest vast amounts of internal and external data (e.g., contracts, invoices, supplier audits, regulatory filings) to flag anomalies or potential compliance risks. Generative AI pushes this even further by translating complex legalese into plain English, generating audit reports, and suggesting tailored mitigation plans based on jurisdiction or industry.
For instance, TraceGrains recently launched an Intelligent Document Processing (IDP) solution for material compliance and lot-level ingredient quality control. Built on OpenAI’s foundation model and trained on global food and beverage industry data, the AI tool streamlines Certificates of Analysis (COAs) reviews. Instead of spending an average of 12 minutes per page on manual checks, users get instant, automated verification — flagging deviations and ensuring documentation aligns with quality, safety, and regulatory benchmarks.
Prewave, meanwhile, built an AI system for tracking and managing over 140 different risk types in global supply chains. These include resilience-related threats like natural disasters, financial instability, and cyberattacks, as well as ESG and regulatory compliance risks.
By continuously scanning news sources, filings, and local reports, Prewave helps companies stay alert to emerging compliance issues across all tiers of their supply network, even in remote or high-risk regions. One of its clients, a pharma company Purem, achieved compliance with German Supply Chain Due Diligence Act and started preparing its operations for the upcoming EUDR, CSRD, and CSDDD regulations.
In supply chain management, AI flips the compliance script. Companies can switch from reacting to violations to proactively mitigating them before they become costly problems. For supply chain leaders, that’s a powerful shift: less firefighting, more foresight.
Conclusion
AI in supply chain management is no longer a bold bet, it’s a proven tactic for improving operational efficiency, risk management, and regulatory compliance, as early adopters have proved.
Predictive systems help cope with the omnipresent market turbulence. GenAI agents and RAG-augmented tools streamline a wide range of knowledge-related workflows and data analysis. Digital twins, AIRO, and decision intelligence systems enable a new pane of real-time, dynamic measurement and management.
If you’re ready to move from AI experimentation to supply chain transformation, Edvantis AI engineering team would be delighted to help you with readiness assessment, use case selection, validation, and implementation. Get in touch with us!