Data has become intrinsic to business operations, fueling innovation, cost-efficiency, and smarter decision-making. Last year, 78% of leaders made good progress with driving innovation with data, 49% are now managing data as a business asset, and 48% have created data-driven organizations, according to a Wavestone survey.
However, most companies are still far from reaching the plateau of data productivity. So in 2025, 82% plan to increase funding in business intelligence and data analytics. But where exactly will those investments go? Edvantis did the market analysis to find out.
- Key Data Analytics Trends for 2025
- Conclusion
Key Data Analytics Trends for 2025
Companies have already migrated most of their data and analytics to the cloud and are now working on improving their processing capabilities. Some 70% of leaders expect that over half of corporate will be on the data lakehouse and 86% also plan to unify analytics data in a central repository.
However, greater data volumes also create new challenges — the risks of ‘data swamping’, poor data visibility, and subsequent silos. Companies still struggle to extract the most relevant and meaningful data from the abundance of raw intel, available to them.
Only one in ten executives say their organizations are “highly mature” in data connectivity and interoperability.
Accenture
Hence, in 2025 we expect that most data analytics investments will be channeled to the next six imperatives:
- Addressing data silos
- Improving data quality
- Implementation of real-time data streaming
- Deployment of LLMs for data analytics
- Data enrichment for better insights
- Compliance with new regulations
The Battle With the Data Silos Continues
Businesses sit on plasters of data, but using these reserves is about as easy as drilling a new oil in real life. Data silos increase proportionally to data volumes, which now grow by about 50% per year. Hence its elimination requires continuous efforts, not just ad hoc tactical initiatives, applied to specific apps or data systems.
On average, 56% of leaders now have to deal with 1,000+ data sources, not to mention an even bigger data management infrastructure estate. So not surprisingly, data silos is the top concern for 68% of business leaders in 2025, up 7% from the previous year.
The solution to data silos is a better, cross-organization data inventory, coupled with data quality metrics and automated monitoring to ensure higher data availability, consistency, quality, and regulatory compliance.
At the most fundamental level, companies need to invest in a data discovery and metadata management platform to create and maintain a data catalog. DataHub, developed by LinkedIn, is a great open-source solution with a metadata service and index appliers written in Java and real-time metadata stream capabilities, written in Python. Microsoft Purview, in turn, is an excellent data discovery platform for the Microsoft ecosystem with extra capabilities for applying unified data security, governance, and compliance.
Implementing automated data governance — a collection of policies and controls for managing data availability, usability, integrity, and security — is the next cornerstone practice. Data governance aims to establish data owners across domains, apply necessary security measures for different types of information, and establish intended uses for each.
Data governance helps build a shared understanding of available data across systems, its origins, sensitivity levels, and authorized use cases for analytics, documented as common data standards, access, and security policies. The data catalog and its supporting documentation then become the foundation for self-service data analytics solutions such as APIs, which enable better data accessibility across the organization.
Data governance platforms like Collibra and Atlan help streamline data governance through the implementation of automated lineage streaking, policy enforcement, data security, and compliance monitoring.
Improving Data Quality and Availability is a Key Priority
Three-quarters of business leaders name “data-driven decision-making” as the top goal of their data programs, yet 67% still don’t completely trust the data they rely on for these decisions.
Lack of trust is a direct result of high data silos and lack of proper data quality controls, making analytics models less reliable. Accurate, consistent, and relevant data is also required for more ambitious AI initiatives. However, only 12% of organizations say that their data is of sufficient quality and accessibility for effective AI implementation. Without the right underlying data, AI model outputs may be useless or, worse, inaccurate or biased. The latter pose major security and compliance risks.
Companies lack readiness in several critical areas for AI adoption
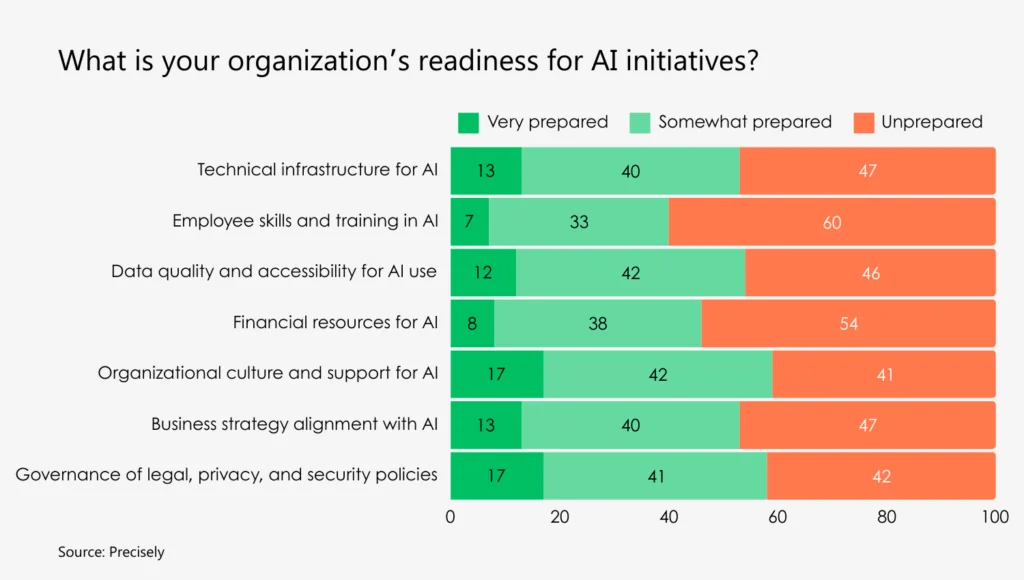
Source: Precisely
One actively discussed solution to the data availability problem is synthetic data — mock, computer-generated data that shares some characteristics of real datasets.
Data scientists can train and validate machine learning and deep learning models on synthetic data before giving them access to limited real-world insights. Synthetic data can also provide exposure to more diverse datasets to compensate for bias and limitations in the real-world ones.
The autonomous vehicle company Waymo uses synthetic data to train algorithms to navigate more complex situations, such as avoiding collisions. In a recent paper, the research team shared that showing the AV model, high-risk, but rare training examples — situations where an accident can happen — increased its accuracy by 15% while only using 10% of the total available training data.
Beyond autonomous driving, scientists also believe that synthetic data can be a better option for training AI models in domains like healthcare or finance, where access to consumer information is limited by regulations.
Additionally, nearly three-quarters of leaders believe that market researchers will be primarily done with synthetic responses within 3 years, according to Qualtrics. Of those, who already used synthetic responses to test packaging, product names, and brand messaging, 87% were satisfied with the results.
Overall, synthetic data could help leaders improve the accuracy of data analytics models, without substantially increasing budgets or compromising on data security. It can also help improve dataset diversity, leading to richer, more relevant insights.
Real-Time Data Streaming Implementation
Traditional data analytics systems rely on pre-scheduled batch ETL/ELT data uploads. Yet, many big data analytics use cases in healthcare or logistics require real-time data streaming aka the ability to continuously ingest, transform, and analyze incoming data with minimal latency.
In retail, real-time data streaming can enable hyper-personalization and dynamic pricing, driven by real-time market signals and user behaviors. Jon Vines, Engineering Lead at AO.com, one of the UK’s largest electrical retailers, shared that data streaming has helped their company create a unified view of each customer, “giving them what they want right at the moment, including product suggestions and relevant promotions to help guide their shopping decisions.”. This hyper-personalization has led to a 30% increase in customer conversion rates.
In healthcare, data streaming enables a new vista of remote patient monitoring scenarios. Edvantis team recently helped SEMDATEX implement a real-time medical data processing system that collects vitals like heart rate, blood pressure, ESG, and oxygen saturation in real-time from connected medical devices and circulates this data across other systems. Healthcare professionals receive instant alerts about changes in patient status, while all collected vitals are automatically added to the patient records and other hospital systems.
Overall, more than three-quarters of enterprises’ standard information architectures will include streaming data and event processing by 2026. Such architectures are primarily built with technologies like open-source Apache Kafka, AWS Kinesis, and Azure Stream Analytics — all great choices in terms of scalability and security. Some of the largest financial institutions including JP Morgan Chase and Goldman Sachs use the Apache framework for real-time financial data analysis, fraud detection, and risk management.
LLMs Deployment for Accessible Data Analytics
Companies increasingly seek simpler interfaces for manipulating complex datasets and visualizing analysis outputs as business users feel intimidated by complex dashboards. Conversational AI interfaces enable companies to make analytical insights more accessible and interpretable.
Large language models (LLMs) like GPT, Gemini, and Claude, among others, can be fine-tuned to perform a variety of data wrangling and modeling tasks such as:
- Query corporate databases with natural language
- Produce custom charts and models on request
- Identify the most recent and relevant information available
- Generate trend predictions, forecasts, and next-best-action recommendations
- Explain complex diagrams, correlations, and outliers in modeled data
In other words, LLMs allow users to interact with analytics without calling upon business analysis or IT teams to set up a new reporting view. This, in turn, allows companies to operationalize more dormant data without spending extra expensive BI licenses or allocating extra human resources.
Pharma company Bayer recently adopted Cortex Analyst — a fully-managed LLM, powered by Snowflake generative AI engine. Previously, the Bayer team could only access enterprise data platform via pre-made dashboards, which often lacked the flexibility to address different use cases and had limited query concurrency. With Cortex Analyst, anyone can query available data in natural language. For example, “What was the market share of product X over the last month?” or perform deeper row-level analysis with less friction.
DeepSearch Labs Team, in turn, developed a gen AI app for investment analysis and company due diligence for a European VC fund. The application has saved the firm’s analysis hours on situational and space awareness analysis, which requires assessment of the market size, growth drivers, trends, competition, and key market players.
The advantage of LLMs for data analytics is that you can build a swarm of data agents to support a variety of data extraction and assistive reasoning tasks to streamline complex problem-solving.
Data Enrichment is in Focus
Companies with more mature data and analytics programs are looking to bring more intel from third parties this year to improve the quality and completeness of their models. Data enrichment from third-party sources — firmographic firms, data brokers, geospatial data providers, and market research firms — can fill in the gaps in incomplete data sets and provide a more representative view of the model scenarios.
Data enrichment enables:
- 360-degree customer view
- Customer identity conflict resolution
- Better ad targeting and personalization
- High-precision campaign attribution
- Enhanced lead scoring
- Improved fraud detection
- Deeper market research
Bloomberg, for example, launched a new data enrichment tool for API-based access to its vast financial markets data. It includes access to full trade life cycle data and benchmark data, showing grouping for the executed trades. With Data Access, customers can enter complex queries to perform trend analysis of broker and trader performance and integrate it with data visualization tools.
In real estate data analytics, data enrichment brings extra location intelligence, climate insights, and neighborhood demographic trends, allowing more accurate valuations and comparables estimates. For instance, Edvantis helped KPC Labs deploy a new data analytics engine, which combines internal and third-party data sources for predicting likely-to-list (likely-to-sell) off-market properties and home valuation ranges.
In the logistics sector, enrichment with geospatial data enables better route planning and real-time optimization. Access to datasets for address verification, geocoding, and location intelligence reduces delivery errors, lowers fuel consumption through better planning, and improves delivery ETAs. Live location and traffic data integration also enables dynamic route adjustments for more effective resource usage as well as geofencing capabilities for greater cargo safety.
Businesses Will Have to Face More Complex Regulations
Compliance continues to remain a common barrier to wider data analytics and AI usage. The global regulatory landscape is largely fragmented with governments and privacy watchdogs taking different approaches to regulations.
In 2025, the era of ‘free reign’ AI will be coming to an end in the EU as the AI Act comes into effect. Starting from February 2025, its enforcement on prohibited use cases — including social scoring, biometric categorization, emotion recognition, predictive policing, and untargeted data scraping from public sources — will kick off. By June 2025, provisions will expand to general-purpose AI (GPAI) models.
In the UK, on the other hand, the new Data (Use and Access) Bill, introduced on 23 October 2024, contains provisions to relax rules around the use of AI in automated decision-making. However, it still requires data controllers within organizations to ensure “that safeguards for the data subject’s rights, freedoms and legitimate interests are in place”. These include the ability to provide users with information about the AI decisions, and the ability to secure human intervention when needed or contest them.
In the US, uncertainty around AI regulations on the federal level looms post-election. State-wise, some 700 AI-related bills were submitted through 2024 and many have a chance to become laws through 2025. Overall, US lawmakers are looking to regulate high-risk AI uses, digital replicas (deepfake) proliferation, and governance use of AI.
Colorado was the first state to enact a comprehensive AI bill, which will come into effect on February 1, 2026. It applies to both AI system developers and deployments (those who are using such solutions) operating in Colorado. Both groups must implement reasonable measures to protect consumers from algorithmic discrimination and disclose the use of high-risk AI systems to consumers, informing them about their rights concerning data processing and decision-making. AI developers must also submit detailed documentation regarding the proposed AI system(s), covering intended uses, performance evaluations, and measures taken to mitigate discrimination risks.
With higher regulatory pressure, proper data governance and AI system explainability will become even more crucial.
Conclusion
Horizontal data analytics integration into more processes and company divisions will hold the top position on the leaders’ agenda through 2025. To achieve that goal, most operators will continue to invest in better data governance solutions to improve data accessibility, availability, and quality, while also expanding their data catalogs with synthetic and third-party data via enrichment services.
We also expect an increased focus on transforming legacy data architectures to support real-time streaming and greater user concurrency. Data mesh and reverse ETL, as well as fine-tuned LLM networks will be the key technologies, helping with that. The adoption of new data analytics and AI solutions will be also guided by emerging regulations, with an increased focus on data traceability, model explainability, and security.
For more insights and strategies on how to act on emerging data analytics trends, reach out to the Edvantis data science team. We’d be delighted to help you determine the key priorities to achieving long-term value with your analytics program. Contact us!