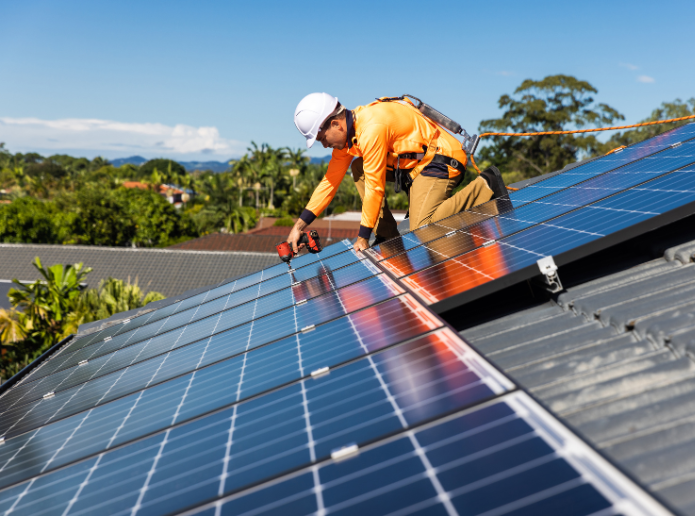
The call for more sustainable power generation has been loud and clear for several years. Net-zero targets and ongoing geopolitical tensions have prompted legislators to pressure businesses to transition to green energy production. At the same time, energy consumption levels also grow, demanding leaner operations.
To accelerate the transition from traditional energy production to digital and sustainable energy generation, business leaders need to invest in new technologies: cloud computing, predictive analytics, digital twins, and IoT among other sectors. Learn how digital transformation in the energy sector takes shape from this post.
6 Digital Transformation Opportunities in the Energy Sector
Between now and 2030, electricity consumption in the EU is expected to increase by 60%. Given the current progress, the U.S. Energy Information Administration (EIA) is concerned that the global energy consumption increases may outpace efficiency gains and drive continued emissions growth by 2050.
These factors will likely translate to extra regulatory pressure on the energy companies to increase the production of renewable energy and emission offsetting. To cope with the task, leaders are making investments in the following digital technologies.
Successfully transform your business with a modern digital ecosystem.
1. Data-Driven Distributed Grid Management
Since 2015 grid-related investment in digital technologies has grown by over 50%, according to IEA. In 2023, it reached 19% of the total grid investment, with the distribution segment commanding 75% of the total digital spending. By modernizing the distribution segment through the integration of smart technologies, leaders can achieve better demand management and grid stability.
Distributed energy systems (DES) offer several advantages over centralized energy systems. They are more versatile and can employ a wide range of energy resources and technologies to be grid-connected or off-grid. In fact, most of the off-grid DESs are renewables-based, proving more economically competitive in remote communities than conventional energy systems. Grid-connected solutions, in turn, can supply the extra capacities during peak demand flexibly and sustainably.
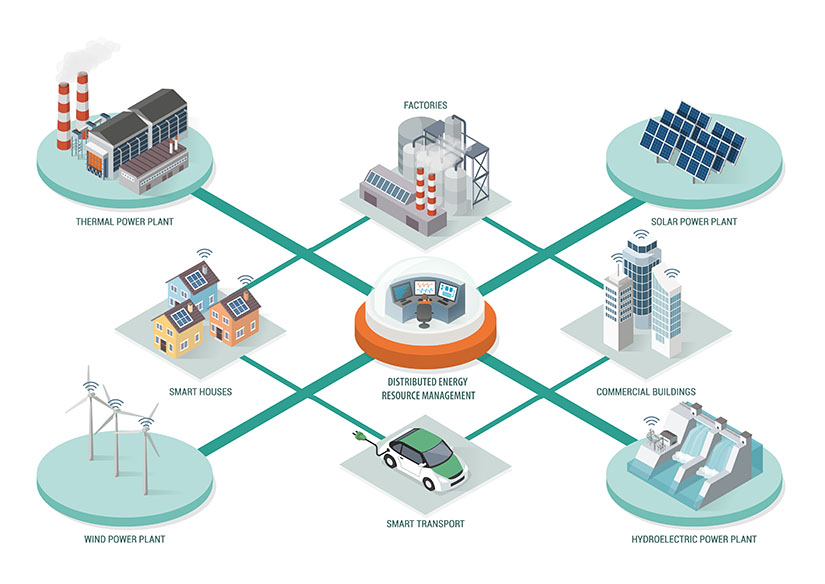
Source: NREL
Although DES can dramatically help with supply and distribution, it also poses new challenges for energy companies. Because DER can generate, store, or flexibly draw or discharge power to the grid, the demand is becoming increasingly variable, impeding grid balancing. Likewise, renewable energy generation levels can be unpredictable too due to weather, which complicates capacity planning.
Investment in digital technologies like cloud, IoT, and big data analytics, can help businesses improve DES management through the following capabilities:
- Forecast management. A data analytics solution can be placed between the network management systems (which provide real-time controls), and planning suites (which help prepare for long-term changes to the network). Forecast analytics systems will allow us to better estimate short-term peaks in demand and model the necessary grid transformation to account for future demand growth.
- Demand-side management. Thanks to the wider adoption of IoT (smart meters, remote monitoring systems, condition monitoring solutions, smart inverters, etc), power companies gain greater visibility into demand and supply. This allows more granular controls. For example, water heaters can be switched on when photovoltaic energy production increases the voltage of the LV networks in residential areas.
- Battery charging infrastructure management. As more electric vehicles (EVs) hit the road, new solutions are required for managing various battery-charging methods (fast, slow, or scheduled). Leaders should also plan for managing battery-based storage, which could play an important role in the networks as capacities increase.
EDF International Networks, for example, is working together with Capgemini on accelerating global deployments of smart grids — grids that use digital technologies for real-time monitoring and optimized distribution. On the home turf in France, EDF helped RTE and Enedis, France’s TSO and DSO, integrate a number of smart grid solutions into their day-to-day network management process. According to the global Smart Grid Index, Enedis was ranked first for the second year in a row in 2023.
Abroad EDF has been involved in launching a microgrid demonstrator in Singapore and smart grid deployment in Uruguay. The current state of technologies already makes advanced network automation possible, so leaders must act on the available opportunities.
2. AI-Powered Energy Forecasting
Smart grids generate massive quantities of data, which contain insights into better management. The global wind turbines produce over 400 billion data points per year. To transform the incoming firehouse of data into operational insights, energy sector leaders are turning to machine learning (ML) and artificial intelligence (AI).
According to Gartner, 92% of power and utility companies plan to incorporate AI and ML into their operations by 2026.
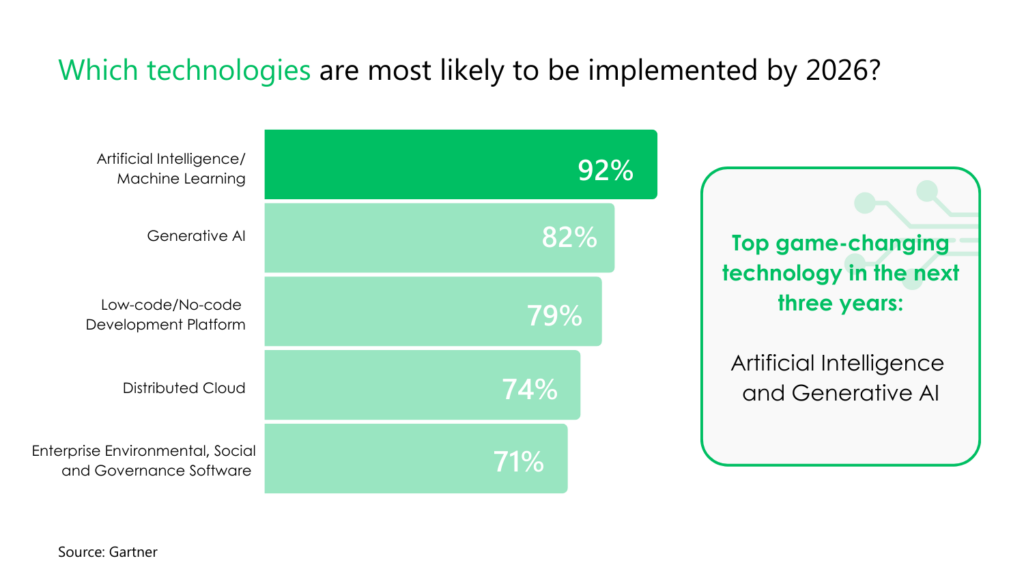
Source: Gartner
One area where AI is already making tangible impacts is supply and demand forecasting. Hydro-Québec (HQ) in Canada operates some 60 hydroelectric generating stations, supplying the region with green energy. Short-term load demand forecasting (a few hours to a few days horizon) is critical to ensure proper generation management, reliability, and maintenance of the power grid.
Since 2018, HQ has developed a range of AI-based load demand forecasting models. The suite of AI tools allows the company to produce load forecasting models from both a macroscopic (also referred to as “top-down”) and microscopic (known as “bottom-up”) point of view. Macroscopic data uses aggregated consumption data from power meters in the province. Microscopic data comes from millions of smart meters.
Using the system, HQ can evaluate the impacts of load, weather, market changes, and other factors to build accurate forecasts, perform congestion analysis, schedule maintenance, and ensure adequate power generation and transmission at all times.
Google DeepMind, in turn, created an AI model to improve the accuracy of forecasts for its 700 MW renewable fleet of wind turbines. Using historical data, the team trained a neural network to predict future energy outputs 36 hours in advance. This way, Google can sell its spare power ahead of time, rather than in real time. By leveraging AI predictions, it has increased the financial value of its wind power by 20%. Enticed by the results, Engie partnered with Google to optimize its wind portfolio using AI.
AI-provided insights also allow energy companies to implement better tariffs, benefiting the consumers. Swiss ABB launched an AI-enabled energy demand forecasting application for commercial building managers that allows them to optimize usage in real time to avoid peak charges and benefit from time-of-use tariffs.
Generative AI is also making inroads in the industry. Researchers from MIT Lincoln Laboratory are testing a gen AI application for smart grid modeling. Using a HILLTOP+ microgrid simulation platform, the team plans to test new smart grid technologies (hardware and software) in a virtual environment first to better understand the system scalability and interoperability before physical deployment.
3. Predictive Maintenance
With consolidated, digital data from a combination of grid assets, energy companies can enable predictive maintenance scenarios.
Using data analysis and machine learning, predictive maintenance systems identify potential equipment failures before they occur using real-time conditioning data. With greater visibility into the asset condition, operators can schedule just-in-time interventions, ensuring that maintenance is carried out when necessary. Such an approach helps maximize the equipment’s lifespan and performance, as well as minimize downtime and maintenance costs.
Italian Enel S.p.A. relies on several predictive maintenance systems across its portfolio of hydroelectric, thermoelectric, nuclear, geothermal, wind-power, and photovoltaic power stations. At a thermoelectric plant in Brindisi, the company rolled out predictive maintenance systems on some 450 critical machines. This has reduced incidents by up to 90% in some applications, preventing a downtime of $366,000 in one case.
Siemens went a step further and recently added gen AI capabilities to its Senseye Predictive Maintenance platform. The platform already incorporates AI and ML algorithms to auto-detect equipment performance issues and alert users. With new Gen AI features, technicians can also request further information from the system using text commands to get extra clarifications and cross-correlate data. The interactive dialogue will speed up and streamline the decision-making process.
4. Digital Twins
Digital twins are data-based, digital replicas of real-world assets. They can represent a solar farm or a hydrogen manufacturing facility, visualizing all the processes and their outputs in the facility.
Effectively, a digital twin aggregates and transforms the available structured (database entries, reports, real-time machinery data streams) and unstructured data (print documents, images, audio, etc) into a real-time model of the asset or facility. Real-time visibility can help operators diagnose potential issues earlier, optimize operational efficiencies, and evaluate new operating scenarios.
E.ON Group, for example, developed a digital twin platform to aggregate and visualize data from its portfolio of power transformers. Since equipment originated from different manufacturers and distribution grids were managed by different regional operators, asset condition data from the transformers was not centrally available for analysis.
The new platform helps teams visualize the location of all transformers, and review individual set characteristics and time-series data from a web dashboard. Furthermore, users can easily evaluate failure modes per asset type using assessment functions to determine the remaining life of the equipment and prioritize capital investments.
German Next Kraftwerke went a step further and virtualized its end-to-end operation. The company has combined data from over 10,000 assets into one of Europe’s largest virtual power plants (VPP) installations. Using the Next Kraftwerke platform, users can obtain accurate supply and demand forecasts to better balance renewable power sources and participate in trades.
Tokyo Electric Power Company (TEPCO), in turn, launched a virtual power plant project in 2019. Its VPP aggregates data from solar, PV, and battery-based sources and validates it against the demand response for optimized energy supply and demand. The project has helped TEPCO improve grid stability and integrate DES sources into the grid.
5. Electric Vehicle Charging Infrastructure
Some 40 million EVs are already on the roads and by 2030, the global EV stock will increase to 350 million vehicles, according to IEA. Electric fleet growth calls for better EV charging infrastructure, which is currently in short supply. The US alone will need over one million extra public EV chargers by 2030 to support federal EV adoption targets.
Energy companies can capitalize on the demand for charging infrastructure by moving from being just energy distributors to service suppliers. For example, they can provide public, overnight charging. TotalEnergies already does this in the Netherlands, supplying 100% renewable, locally-generated power to consumers.
EDF and Nissan launched a new commercial vehicle-to-grid (V2G) service for corporate EV fleets in the UK. The service supports two-way energy flows, recharging the EV battery when the tariffs are the lowest and discharging excess energy to sell back into the grid. Oncor Electric Delivery and Toyota Motor North America are also collaborating on a V2G pilot in the region.
Effectively, energy players can access new revenue streams by collaborating with the automotive sector on EV charging solutions to further incentivize adoption. After all, EVs can become a new element of the distributed energy systems, participating both in demand and supply. Enel partnered with Nissan in Denmark to promote energy trading among EV owners. When using the ‘smart charging’ feature on its infrastructure, fleet owners will receive rebates for assisting the grid.
6. Peer to Peer Trading
As more grinds become decentralized, we’re seeing a new wave of prosumers – individuals who both consume and produce energy. These may include EV owners, as well as households with solar panel installations or businesses, operating wind farmers. All of them can generate, store, and trade produce energy in a decentralized fashion using a peer-to-peer (P2P) marketplace. P2P electricity trading schemes are already underway in Australia, Colombia, Germany, Japan, Malaysia, the Netherlands, the UK, and the US.
P2P energy trading platforms like PowerLedger, Exnaton, and ENTRNCE take the traditional role of an energy retailer by managing the price and volume of buy-sell transactions. Supported trade scenarios include over-the-grid trading, when the prosumer is connected to the central grid, but manages price and volume risk independently through direct peer trades. The Vandebron platform allows consumers to purchase power directly from prosumers who set the price. It balances the wholesale markets by connecting consumers, prosumers, and generators, plus provides suppliers with generation forecasts for their assets.
In a partly independent microgrid scenario, the prosumer creates a microgrid that manages some of the aggregate energy needs but remains connected to the central grid for the remainder. One example of this setup is the Schoonschip Amsterdam microgrid. In this community-led project, the surplus electricity is traded among its members, but the community remains grid-connected for redundancy.
The Port of Rotterdam also ran a similar experiment. During the pilot, commercial consumers could trade renewable energy via the decentralized platform with the help of an AI-enabled energy trading agent, which suggested procurement strategies, based on their needs. The results were rather impressive: consumers reduced costs by 11%, while producers gained a 14% increase in revenue. Additionally, during the trial 92% of solar-generated energy was consumed, overcoming historic losses.
The third possible trading scenario is a fully independent microgrid — a privately operated, self-sufficient network, not connected to any central electricity network, which supports intra-participant P2P trades. The Brooklyn Microgrid project is working towards this vision. Led by LO3 Energy with support from Siemens, the team is working on an independent community energy market. The microgrid combines network control systems, switchgear, innovative battery solutions, and smart electric meters, which help with load balancing and demand forecasting. Participants can buy and sell energy using smart contracts based on blockchain.
For energy companies, P2P trading presents yet another avenue to incentivize decentralized production, generate a profit on trading transactions, and improve supply to underserved communities.
Conclusion
The energy market is on the brink of major transformations. Decentralized energy production and P2P trading will grow in volumes, incentivized by government support. EV fleet owners, in turn, will further expand the DES market as V2G technology becomes more widely deployed. By choosing the path of collaboration, energy companies can accelerate their transition to more sustainable energy production and share some of the capital expenditures on infrastructure.
Investments in digital technologies, in turn, will help effectively integrate DES distribution into existing network flows to better forecast demand, optimize production volumes, and optimize prices. Edvantis team would be delighted to further advise you on how to approach digital transformations. Contact us for a consultation.